AI/ML Mastery
Harnessing the Power of Artificial Intelligence and Machine Learning
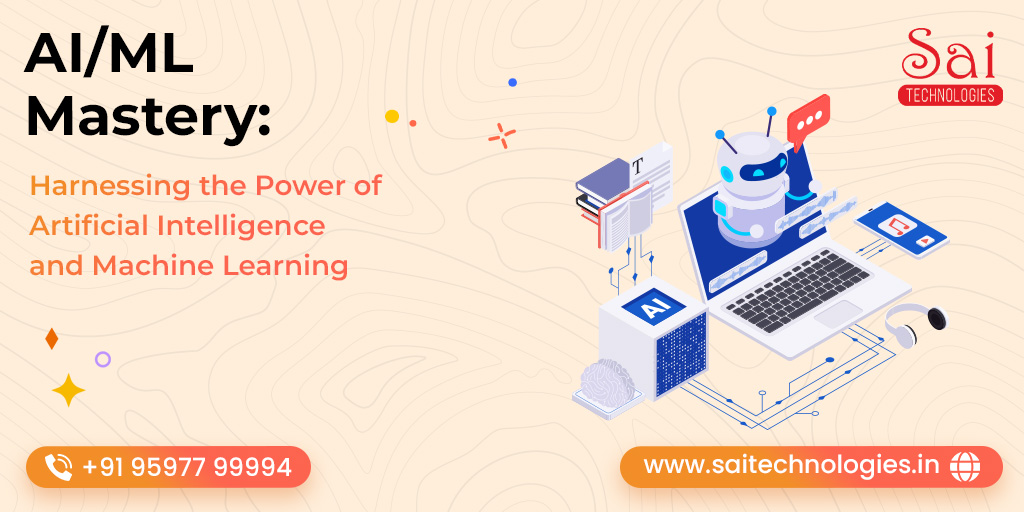
Artificial intelligence (AI) and machine learning (ML) have emerged as revolutionary technologies with the potential to change industries, restructure business models, and spur innovation in today's quickly expanding digital ecosystem. AI and ML algorithms are powering a wide range of applications across varied fields, from autonomous systems and natural language processing to personalized recommendations and predictive analytics. In order to help enterprises take advantage of new opportunities, streamline operations, and achieve a competitive edge in the digital age, we will dive into the nuances of AI/ML mastery in this blog.
Understanding Artificial Intelligence and Machine Learning
Artificial intelligence encompasses a broad range of techniques and methodologies aimed at creating systems that can perform tasks traditionally requiring human intelligence. Machine learning, a subset of AI, focuses on developing algorithms that can learn from data, identify patterns, and make predictions or decisions without explicit programming. Understanding the fundamentals of AI and ML is essential for leveraging their potential effectively.
Leveraging AI/ML for Predictive Analytics
Predictive analytics leverages AI and ML algorithms to analyze historical data, identify trends, and forecast future outcomes or behaviors. By extracting insights from large datasets and identifying hidden patterns, organizations can anticipate market trends, optimize operations, and make data-driven decisions with greater confidence and accuracy.
Personalization and Recommendation Systems
AI-powered recommendation systems use ML algorithms to analyze user preferences, behavior, and interactions to deliver personalized content, product recommendations, and services. By understanding individual preferences and anticipating user needs, organizations can enhance customer experiences, drive engagement, and increase conversion rates across various digital platforms.
Natural Language Processing and Conversational AI
Natural language processing (NLP) enables computers to understand, interpret, and generate human language, facilitating communication between humans and machines. Conversational AI applications such as chatbots, virtual assistants, and voice-enabled interfaces leverage NLP algorithms to provide personalized assistance, automate customer service interactions, and streamline workflows in various industries.
Autonomous Systems and Robotics
Autonomous systems and robotics integrate AI and ML technologies to enable machines to operate independently, make decisions, and adapt to changing environments. From autonomous vehicles and drones to industrial robots and smart appliances, autonomous systems are revolutionizing industries, increasing efficiency, and reducing human intervention in repetitive or hazardous tasks.
Deep Learning: Unraveling Complex Patterns
Deep learning, a subset of ML, involves training artificial neural networks with multiple layers to recognize patterns and extract features from large datasets. Deep learning algorithms have achieved remarkable success in image recognition, speech recognition, and natural language processing tasks, enabling breakthroughs in areas such as healthcare diagnostics, autonomous driving, and financial forecasting.
AI Ethics and Responsible AI Deployment
As AI and ML technologies become increasingly pervasive, ensuring ethical and responsible deployment is paramount. Organizations must consider factors such as fairness, transparency, accountability, and privacy to mitigate potential biases, risks, and unintended consequences associated with AI systems. Ethical AI frameworks and guidelines help promote trust, fairness, and inclusivity in AI-powered solutions.
Reinforcement Learning and Autonomous Decision-Making
Reinforcement learning (RL) enables machines to learn optimal decision-making strategies through trial and error interactions with their environment. Advanced RL algorithms, such as deep Q-networks (DQN) and policy gradient methods, have applications in autonomous systems, robotics, and game playing, where agents learn to maximize long-term rewards by exploring and exploiting different actions.
Generative Adversarial Networks (GANs) and Creative AI
Generative adversarial networks (GANs) consist of two neural networks, a generator and a discriminator, competing against each other to generate realistic synthetic data samples. GANs have applications in generating photorealistic images, synthesizing music, and creating virtual environments, pushing the boundaries of creative AI and artistic expression.
Transfer Learning and Domain Adaptation
Transfer learning techniques enable AI models to leverage knowledge learned from one task or domain to improve performance on a related task or domain with limited labeled data. Domain adaptation methods, such as adversarial domain adaptation and self-supervised learning, facilitate model adaptation to new environments, datasets, or domains, reducing the need for extensive retraining.
Multi-Agent Systems and Collaborative AI
Multi-agent systems (MAS) involve multiple AI agents interacting with each other and their environment to achieve collective goals or solve complex problems. Collaborative AI frameworks, such as cooperative reinforcement learning and multi-agent deep reinforcement learning, enable agents to learn to collaborate, coordinate, and communicate effectively, leading to emergent behaviors and solutions beyond individual capabilities.
Meta-Learning and AutoML
Meta-learning algorithms aim to learn efficient learning strategies or meta-policies that enable models to adapt quickly to new tasks or environments with minimal data. AutoML frameworks automate the process of model selection, hyperparameter tuning, and architecture search, accelerating the development and deployment of AI models while reducing the need for manual intervention and expertise.
Explainable AI (XAI) and Model Interpretability
Explainable AI (XAI) techniques aim to enhance the transparency and interpretability of AI models, enabling users to understand and trust model predictions and decisions. Model interpretability methods, such as feature attribution, saliency maps, and model-agnostic explanations, provide insights into how models make predictions, helping to identify biases, errors, and potential ethical concerns.
Federated Learning and Privacy-Preserving AI
Federated learning enables collaborative model training across distributed data sources while preserving data privacy and confidentiality. By training models locally on user devices or edge devices and aggregating model updates centrally, federated learning allows organizations to leverage decentralized data without compromising individual privacy or data security, paving the way for privacy-preserving AI applications in healthcare, finance, and IoT.
Quantum Machine Learning and Quantum AI
Quantum machine learning (QML) explores the intersection of quantum computing and machine learning, leveraging quantum algorithms and hardware to solve optimization and pattern recognition problems more efficiently. Quantum AI extends QML concepts to develop AI algorithms and models that exploit quantum properties, such as superposition and entanglement, to perform computations beyond classical limits.
Robust AI and Adversarial Robustness
Robust AI techniques aim to enhance the resilience of AI models against adversarial attacks, data perturbations, and distributional shifts. Adversarial training, adversarial defense mechanisms, and certified robustness guarantees are used to improve model robustness and generalization performance, ensuring reliable and trustworthy AI systems in real-world applications.
Ethical AI Governance and Responsible AI Deployment
Ethical AI governance frameworks provide guidelines and best practices for ensuring responsible and ethical deployment of AI systems. Principles such as fairness, transparency, accountability, and inclusivity guide organizations in designing, implementing, and monitoring AI applications to mitigate biases, promote equity, and uphold societal values and human rights in the development and use of AI technologies.
Overcoming Challenges in AI/ML Implementation
Implementing AI and ML initiatives requires overcoming various challenges, including data quality issues, talent shortages, regulatory compliance, and organizational resistance. Addressing these challenges involves establishing robust data governance practices, investing in talent development and reskilling programs, and fostering a culture of innovation and collaboration across the organization.
Future Trends and Opportunities in AI/ML
Looking ahead, AI and ML are poised to continue advancing rapidly, driven by advancements in hardware, algorithms, and data availability. Emerging trends such as federated learning, explainable AI, and AI-driven automation are reshaping the landscape of AI/ML applications, opening up new opportunities for innovation and value creation across industries.
Building AI/ML Competency and Expertise
Building AI/ML competency requires a combination of technical expertise, domain knowledge, and continuous learning. Organizations can cultivate AI/ML talent through training programs, workshops, and hands-on projects, fostering a culture of experimentation and knowledge sharing to drive innovation and stay ahead in the competitive marketplace.
Real-Time Example: Autonomous Vehicles in Action
The deployment of autonomous vehicles exemplifies AI/ML mastery in the automotive industry. Companies like Tesla, Waymo, and Uber are leveraging AI algorithms for real-time perception, decision-making, and control to navigate vehicles safely and efficiently on public roads. With advancements in computer vision, sensor fusion, and deep learning, autonomous vehicles are poised to revolutionize transportation, reducing accidents and congestion while increasing mobility for passengers.
Industry Insights: AI in Healthcare Diagnostics
AI/ML technologies are transforming healthcare diagnostics by enabling more accurate and efficient disease detection and diagnosis. For example, IBM's Watson for Oncology uses natural language processing and machine learning to assist oncologists in identifying personalized treatment options for cancer patients. According to a survey by the Healthcare Information and Management Systems Society (HIMSS), 73% of healthcare organizations report positive outcomes from AI/ML adoption, including improved diagnostic accuracy and patient outcomes.
Trend Analysis: Rise of AI-Powered Chatbots
The proliferation of AI-powered chatbots across industries reflects a growing trend towards conversational AI and automated customer service. According to Grand View Research, the global chatbot market is projected to reach $1.25 billion by 2025, driven by demand for personalized customer experiences and cost-effective service delivery. Companies like Amazon, Google, and Apple are investing in virtual assistants such as Alexa, Google Assistant, and Siri, which leverage AI/ML to understand user queries, provide relevant information, and perform tasks through natural language interaction.
Statistical Insights: Impact of AI/ML on Business Productivity
Statistics from a McKinsey Global Institute report reveal that AI/ML technologies have the potential to increase global business productivity by up to 1.2% annually. Organizations adopting AI/ML solutions experience significant improvements in operational efficiency, workforce productivity, and innovation capacity. For example, a survey by PwC found that 54% of businesses using AI/ML reported revenue growth, with 60% attributing increased profitability to AI-driven insights and decision-making.
Survey Findings: AI Adoption and Investment Trends
A survey conducted by Deloitte indicates that AI adoption is accelerating across industries, with 73% of executives investing in AI technologies to gain a competitive advantage. Key areas of AI investment include predictive analytics (43%), machine learning (40%), and natural language processing (38%). Moreover, 82% of surveyed executives believe that AI will be "critically important" for their organization's success in the next two years, highlighting the strategic significance of AI/ML mastery in driving business growth and innovation.
Mastering the power of artificial intelligence and machine learning holds the key to unlocking new possibilities, driving innovation, and staying ahead in the digital economy. By understanding the fundamentals, leveraging advanced techniques, and embracing ethical practices, organizations can harness the full potential of AI/ML to transform their operations, deliver personalized experiences, and create value for stakeholders in an increasingly AI-driven world.