Data Science Insights
Leveraging Big Data for Informed Decision-Making
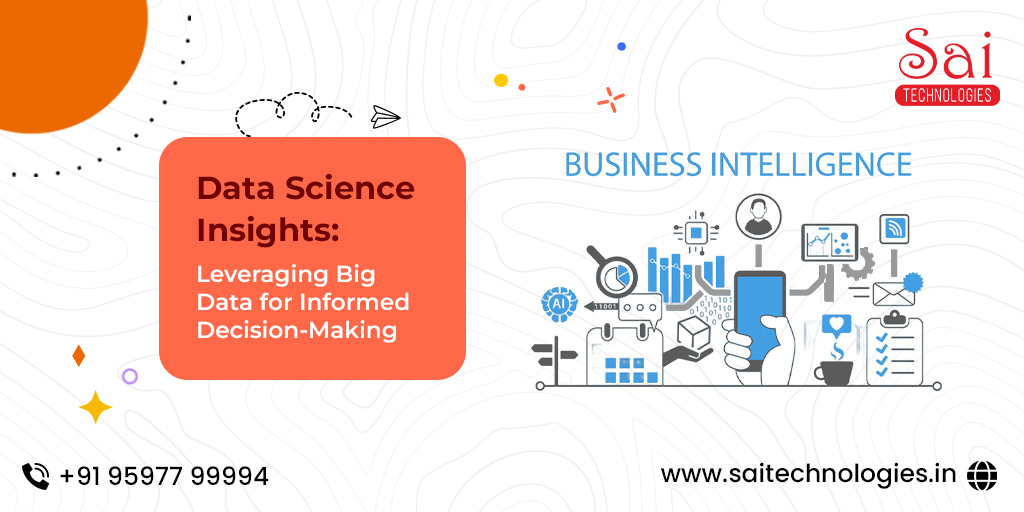
In today's data-driven world, businesses across industries are recognizing the transformative power of big data and data science in driving informed decision-making. From optimizing operations to enhancing customer experiences, leveraging data insights has become essential for staying competitive in the modern landscape. This blog delves into the realm of data science, exploring how organizations can harness big data to gain valuable insights and make informed decisions that drive success.
Understanding Big Data and Data Science:
Definition of Big Data: Big data refers to large and complex datasets that cannot be processed using traditional data processing techniques.
Role of Data Science: Data science encompasses the methods, tools, and techniques used to analyze and extract insights from big data, including machine learning, statistical analysis, and data visualization.
The Importance of Data-Driven Decision-Making:
Enhanced Insights: Big data provides organizations with a wealth of information that can offer deep insights into customer behavior, market trends, and operational performance.
Competitive Advantage: Organizations that leverage data science gain a competitive edge by making informed decisions based on data-driven insights rather than relying on intuition or guesswork.
Improved Efficiency: Data-driven decision-making streamlines processes and operations, leading to increased efficiency and productivity across the organization.
Applications of Data Science in Various Industries:
Retail: Retailers use data science to analyze customer purchase patterns, optimize pricing strategies, and personalize marketing campaigns to enhance customer experiences and drive sales.
Healthcare: In healthcare, data science enables predictive analytics for disease diagnosis, patient monitoring, and personalized treatment plans, leading to improved patient outcomes and cost savings.
Finance: Financial institutions leverage data science for risk management, fraud detection, and algorithmic trading to make more accurate and timely decisions in a dynamic market environment.
Manufacturing: Manufacturers use data science to optimize supply chain operations, improve product quality, and predict equipment maintenance needs, leading to cost savings and increased production efficiency.
Key Components of Data Science:
Data Collection: Gathering relevant data from various sources, including structured and unstructured data, is the first step in the data science process.
Data Cleaning and Preprocessing: Cleaning and preprocessing raw data to remove noise, handle missing values, and standardize formats ensure data quality and consistency.
Exploratory Data Analysis (EDA): EDA involves visualizing and summarizing data to identify patterns, trends, and relationships that can inform further analysis.
Statistical Analysis and Modeling: Statistical analysis and modeling techniques, such as regression analysis, classification, and clustering, are used to extract insights and make predictions from data.
Machine Learning: Machine learning algorithms enable computers to learn from data and make predictions or decisions without being explicitly programmed, allowing for automated decision-making and pattern recognition.
Challenges and Considerations in Data Science:
Data Quality: Ensuring data quality and accuracy is essential for reliable analysis and decision-making. Data may be incomplete, inconsistent, or biased, posing challenges for data scientists.
Scalability: Processing large volumes of data requires scalable infrastructure and efficient algorithms to handle computational complexity and resource constraints.
Privacy and Security: Safeguarding sensitive data from unauthorized access and breaches is a critical consideration in data science, particularly in industries like healthcare and finance.
Interpretability: Interpreting and explaining the results of data analysis and machine learning models in a way that is understandable and actionable for decision-makers is a challenge in data science.
Best Practices for Effective Data Science:
Define Clear Objectives: Clearly define the goals and objectives of data science projects to ensure alignment with business priorities and stakeholder expectations.
Collaborate Across Teams: Foster collaboration between data scientists, domain experts, and business stakeholders to leverage diverse perspectives and domain knowledge.
Invest in Data Infrastructure: Invest in robust data infrastructure and platforms that support data collection, storage, processing, and analysis at scale.
Focus on Data Governance: Implement data governance policies and procedures to ensure data quality, integrity, and compliance with regulations and industry standards.
Continuously Learn and Adapt: Data science is an evolving field, so it's essential to stay updated on emerging technologies, methodologies, and best practices through continuous learning and experimentation.
Real-World Examples of Data Science Success Stories:
Netflix: Netflix leverages data science to analyze viewer preferences and behavior, recommending personalized content to subscribers and optimizing its content library to drive engagement and retention.
Amazon: Amazon uses data science for dynamic pricing, product recommendations, and supply chain optimization, delivering a personalized shopping experience to customers and maximizing operational efficiency.
Google: Google applies data science to improve search algorithms, target advertising, and enhance user experiences across its products and services, such as Google Search, Gmail, and YouTube.
Extracting Insights from Diverse Data Sources
Structured vs. Unstructured Data:
Distinguish between structured and unstructured data and understand their respective challenges and opportunities for analysis. Explore how organizations leverage advanced data processing techniques, such as natural language processing (NLP) and sentiment analysis, to extract insights from unstructured data sources such as text, images, and social media.
Real-Time Data Analytics:
Discover the importance of real-time data analytics in today's fast-paced business environment, where timely insights can make or break decision-making processes. Learn how organizations leverage technologies like stream processing, complex event processing (CEP), and in-memory databases to analyze data in real-time and respond to changing market conditions swiftly.
Future Trends in Data Science:
AI and Automation: Advancements in artificial intelligence (AI) and automation are transforming data science, enabling more sophisticated analysis and decision-making capabilities.
Edge Computing: Edge computing brings data processing closer to the source of data generation, reducing latency and enabling real-time analysis and insights at the network edge.
Ethical AI: Ethical considerations around bias, fairness, and transparency in AI and machine learning algorithms are gaining prominence, shaping the future of responsible data science practices.
Quantum Computing: Quantum computing holds the potential to revolutionize data science by solving complex problems and optimizing algorithms that are intractable for classical computers.
Conclusion:
Data science is a powerful tool for organizations seeking to unlock the value of big data and make informed decisions that drive success. By leveraging data science techniques and technologies, businesses can gain valuable insights, optimize operations, and enhance customer experiences across various industries. As data science continues to evolve, organizations must embrace best practices, collaborate across teams, and invest in scalable infrastructure to harness the full potential of data-driven decision-making in the digital age.